Techniques for Efficient AML Data Management
In today's fast-paced financial world, the handling of Anti-Money Laundering (AML) data stands as a critical pillar in maintaining the integrity of our financial systems. While it’s a term often echoed in the corridors of banks and financial institutions, the essence of AML data management extends far beyond mere compliance. It’s about safeguarding the economic framework that billions rely on daily. However, as the volume of financial transactions skyrockets, managing this data efficiently becomes not just a regulatory necessity but a formidable challenge.
Picture this: each day, millions of transactions flow through the financial network, each a potential clue in the vast puzzle of preventing financial crime. Navigating this high-volume sea of data, with its intricate patterns and hidden anomalies, requires more than traditional methods; it demands innovative techniques and forward-thinking strategies.
In this article, we delve into the world of AML data, unraveling the complexities and revealing the cutting-edge techniques that are reshaping the way financial institutions combat money laundering. Join us as we explore how embracing these techniques can transform challenges into opportunities for safeguarding our financial future.
Understanding AML Data
At the heart of anti-money laundering (AML) efforts lies a crucial component: AML data. But what exactly is AML data? Simply put, it's the information collected and analyzed by financial institutions to prevent and detect money laundering activities. This data plays a pivotal role in the fight against financial crimes, helping to safeguard the integrity of our economic systems.
Types of AML Data
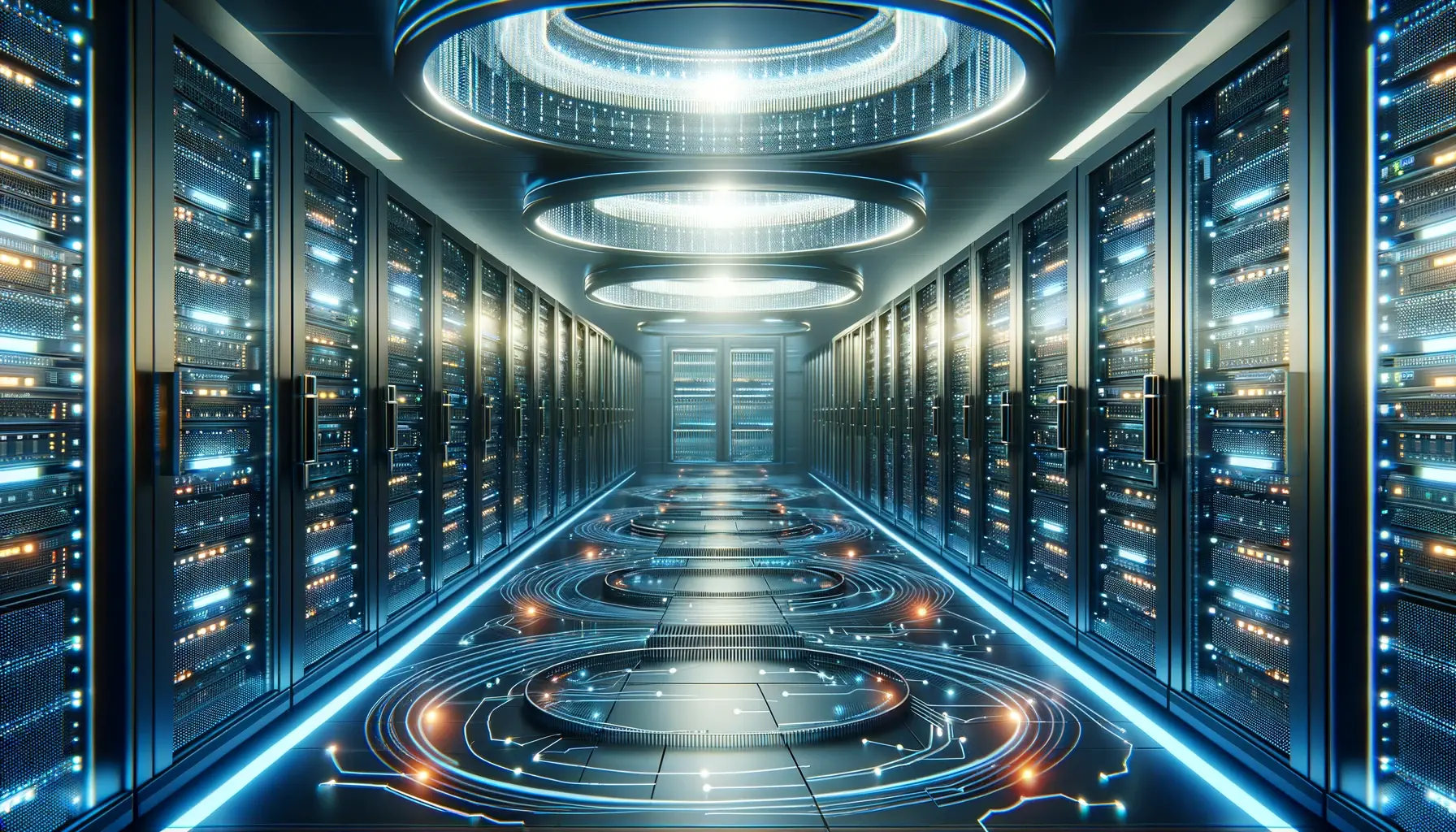
AML data encompasses a wide range of information. Some of the key types include:
- Transactional Data: Records of financial transactions, such as deposits, withdrawals, and transfers. This data helps in identifying unusual patterns that might suggest money laundering.
- Customer Information: Details about customers, including their identity, occupation, financial history, and risk profile. Knowing who is conducting a transaction is as important as the transaction itself.
- Geographical Data: Information on where transactions are occurring, which can be critical, especially if they occur in or involve countries known for higher risks of money laundering.
- Historical Data: Past financial records and behavior of customers. This aids in establishing a baseline against which current transactions can be compared.
Role of AML Data in Compliance and Fraud Prevention
AML data is not just a regulatory requirement; it's a key tool in the proactive prevention of financial fraud. By analyzing this data, financial institutions can:
- Detect Suspicious Activities: Uncover patterns and anomalies in financial transactions that may indicate money laundering.
- Assess Customer Risk: Evaluate the risk level of customers based on their financial activities and background.
- Comply with Regulations: Adhere to legal requirements by monitoring, reporting, and taking necessary actions against suspicious activities.
The Evolving Landscape of AML Data
The world of AML is constantly evolving. With the advent of new technologies and changes in money laundering tactics, the nature of AML data is continuously adapting. Financial institutions must stay updated on these changes to ensure they are effectively analyzing and using AML data to combat financial crimes.
In conclusion, understanding AML data is the first step in developing effective strategies to handle it. By grasping the types of data involved and recognizing its role in compliance and fraud prevention, financial institutions can better equip themselves to tackle the challenges of high-volume AML data management.
The Challenges of High-Volume AML Data
As the volume of financial transactions continues to grow exponentially, managing Anti-Money Laundering (AML) data has become an increasingly complex task. The sheer scale and diversity of this data pose several challenges for financial institutions. Understanding these challenges is essential to develop effective strategies for AML data management.
- Data Complexity and Variety
AML data is not just vast in volume; it's also incredibly diverse and complex. This data comes in various formats and from multiple sources, including domestic and international transactions, customer databases, and third-party sources. The complexity is further compounded by the need to integrate and interpret this disparate data to form a cohesive and accurate picture of customer activities.
- Real-Time Processing Needs
In the realm of AML compliance, time is of the essence. Financial institutions are expected to monitor transactions in real-time or near-real-time to identify and report suspicious activities promptly. This requirement poses a significant challenge in processing and analyzing large volumes of data quickly and accurately.
- Regulatory Compliance Requirements
The regulatory landscape for AML is both stringent and ever-changing. Financial institutions must continuously adapt their AML data management processes to comply with the latest regulations. This involves staying updated on regulatory changes, implementing necessary modifications in data processing, and ensuring complete and timely reporting. Non-compliance can lead to hefty fines and reputational damage.
- Balancing Privacy Concerns
In handling AML data, institutions must also navigate the delicate balance between rigorous monitoring and respecting customer privacy. This is particularly challenging given the sensitive nature of financial data and the increasing concerns and regulations around data privacy and protection.
- Scalability and Flexibility
As financial institutions grow and transaction volumes increase, their AML data management systems must scale accordingly. This scalability needs to be flexible enough to handle fluctuating data volumes and complex data types without compromising on speed or accuracy.
- Integrating Advanced Technologies
With the advancement of technology, there's a growing need to integrate sophisticated tools like AI and machine learning for efficient AML data management. However, implementing these technologies poses its own set of challenges, including the need for skilled personnel, high implementation costs, and ensuring these new systems work seamlessly with existing infrastructure.
Techniques for Efficient AML Data Management
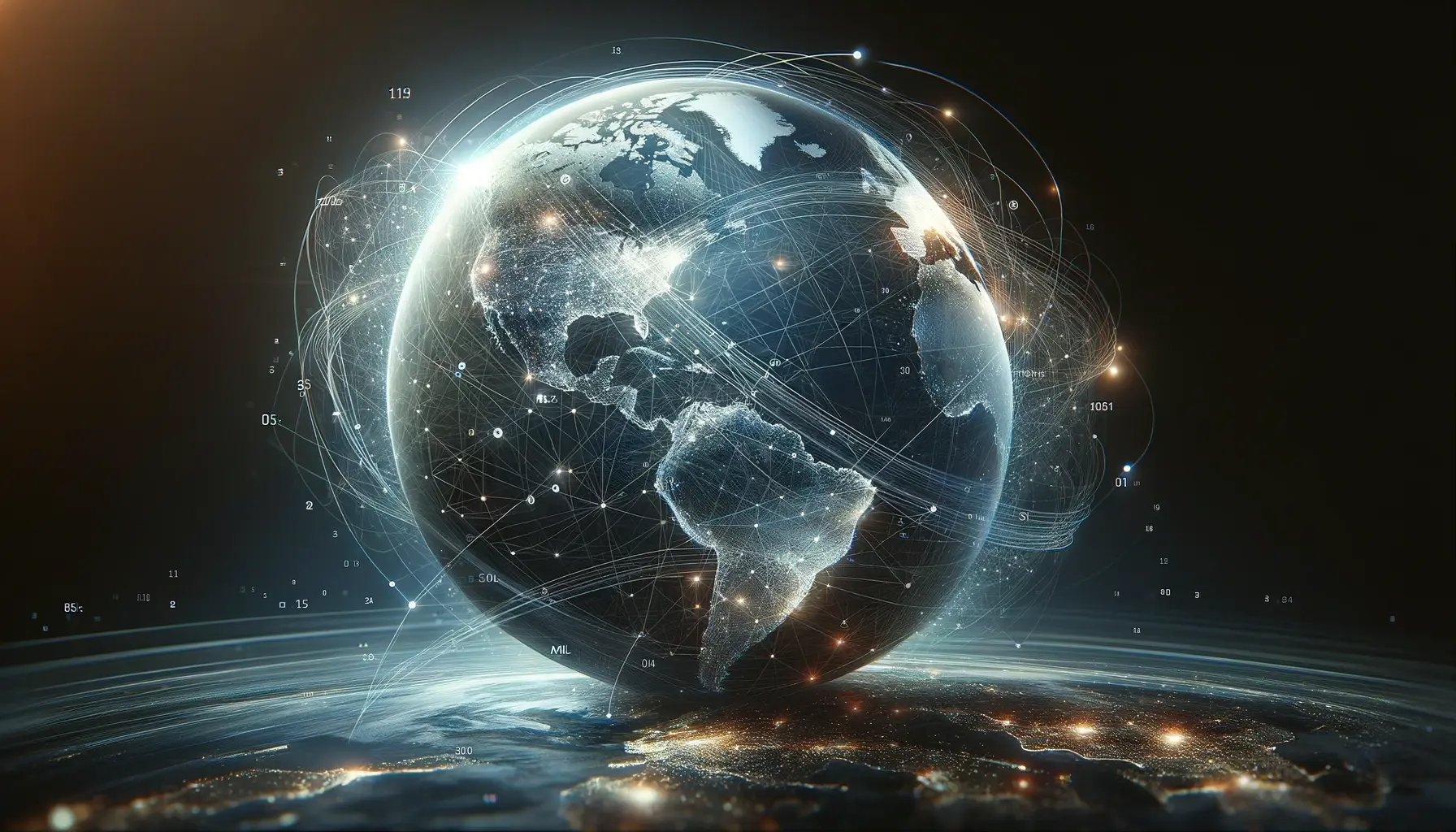
Tackling the challenges of high-volume AML data requires a strategic approach, combining robust processes with advanced technologies. Here, we explore some key techniques that can significantly enhance the efficiency of AML data management in financial institutions.
- Data Integration: Creating a Unified View
A crucial step in efficient AML data management is integrating data from various sources. By consolidating transactional data, customer information, and other relevant data into a single, unified system, institutions can more easily detect suspicious patterns and anomalies. This unified view also aids in comprehensive analysis and faster decision-making.
- Data Quality Management: Ensuring Accuracy and Reliability
The reliability of AML processes heavily depends on the quality of the underlying data. Financial institutions should implement strict data quality management protocols, including regular audits, validation processes, and correction mechanisms. This ensures that the data used for AML monitoring is accurate, complete, and up-to-date.
- Automated Data Processing Systems
Automation plays a key role in handling large volumes of AML data efficiently. Automated data processing systems can rapidly analyze vast amounts of transactions, flagging those that require further investigation. This not only speeds up the process but also reduces the likelihood of human error.
- Implementing Risk-Based Approaches
Adopting a risk-based approach allows institutions to allocate resources more effectively. By categorizing customers and transactions based on their risk levels, institutions can focus their monitoring efforts where they are most needed, improving the overall efficiency of their AML processes.
- Continuous Monitoring and Updating
AML data management is not a one-time task; it requires ongoing monitoring and regular updates. Financial institutions should establish procedures for continuous monitoring of transactions and customer activities, along with periodic updates to their AML strategies and systems in response to evolving threats and regulatory changes.
- Leveraging Advanced Analytics and AI
Advanced analytics and AI technologies can significantly enhance AML data management. These tools can process large datasets more efficiently, identify complex patterns indicative of money laundering, and even predict future risks. However, it's important to balance the use of AI with human oversight to ensure accuracy and compliance.
- Training and Knowledge Sharing
Finally, an often-overlooked aspect of effective AML data management is staff training. Regular training sessions and knowledge-sharing initiatives can help ensure that personnel are up-to-date on the latest AML trends, regulations, and technologies, further enhancing the institution's ability to manage AML data effectively.
Conclusion
The effective handling of AML data goes beyond mere compliance; it's a commitment to uphold the integrity of the financial system and protect it from the perils of money laundering. By embracing a combination of data integration, quality management, automation, risk-based approaches, continuous monitoring, advanced analytics, and ongoing training, institutions can transform AML data management from a daunting challenge into a powerful tool in their arsenal against financial crime.
As we look to the future, it is clear that the landscape of AML data management will continue to evolve, shaped by technological advancements and shifting regulatory environments. Financial institutions that stay ahead of these changes, continuously adapting and innovating, will not only ensure compliance but also contribute significantly to the global fight against money laundering.
In closing, the efficient management of high-volume AML data is not a destination but a continuous journey. It demands diligence, innovation, and a proactive stance from financial institutions. By adopting these principles, they can ensure the safety, stability, and trustworthiness of the financial systems that underpin our economy and society.